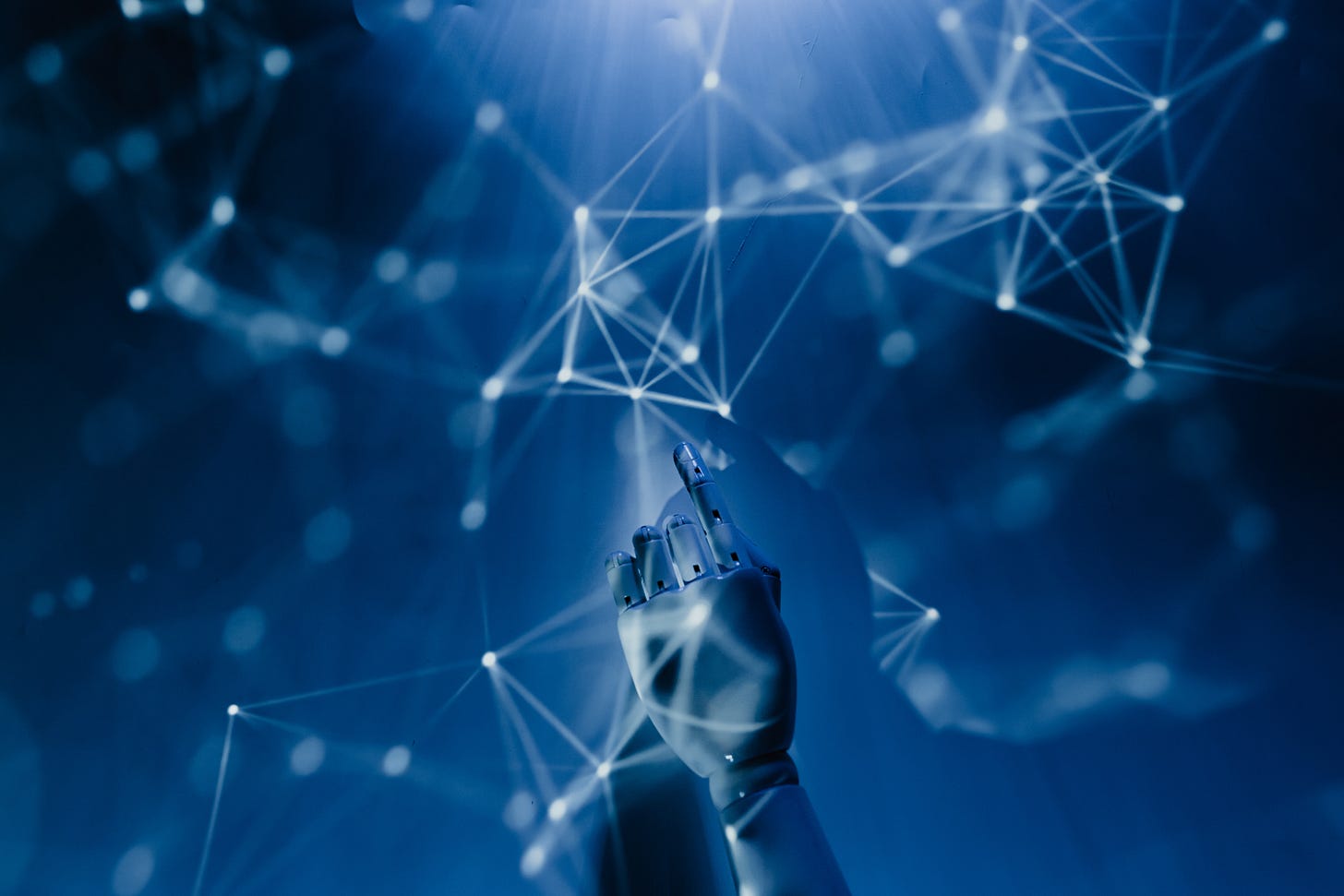
This year, the world got a rude awakening to the insane power of AI when OpenAI unleashed ChatGPT4 onto the world. This AI text generator/chatbot seemed to be able to replicate human-generated content so well that even AI detection software struggled to tell the difference between the two. Overnight, it seemed like any job focused on writing, from copywriters, journalists, screenwriters and even academic writers, was at risk of being made obsolete by a robot, something we didn’t think could happen for decades. However, recent research has found that many of our most powerful AI models, including ChatGPT4, are at serious risk of destabilising themselves to the point of not working, thanks to an AI echo chamber phenomenon. So, can AI save itself from itself?
To understand this problem, we must first understand what AI is and how we make it work. You see, most normal programs are written by coders, and that’s it. But AI uses vast datasets and statistics to write the program rather than a coder (it’s a little more complex than that, but the analogy works fine here).
This process starts with what’s known as training. You get a large dataset of images, videos, text, or raw data and feed it into a neural network. This neutral network uses a similar process to how our brains work to recognise patterns in the dataset. You can then use this “trained” neural network to replicate, identify or predict similar datasets.
So, for example, ChatGPT4 replicates an output with similar patterns to the data it was trained on. There are AIs out there that have been trained on mammogram images and can identify breast cancer before a doctor can. Some AIs can predict if a stock will go up or down in value based on its past data.
This pattern recognition and the ability to replicate it is why AI is so damn powerful. But to make these neural networks work predictably enough to be useful, you need to train them on truly colossal amounts of high-quality data. For example, ChatGPT4 was trained on 570 GB of carefully selected raw text files; that might not sound like a lot, but it is equivalent to 300 billion words! Or, to put it another way, if I wrote flat out 24/7, it would take me 14,270 years to write that number of words.
But where do AI companies get this truly astonishing amount of high-quality data from?
Well, to put it bluntly, they steal it. Medical AIs, stock predicting AIs and more specialist AIs are trained on data that was sold to them, given written permission to use or that they already own. But, for the generative AIs (which aim to replicate more complex things like human writing or images) like ChatGPT4, DALL-E, Midjourney, Google Bard and others, obtaining the training dataset in this way is impractical and eye-waveringly expensive! So instead, they just scrape data off the internet. For example, ChatGPT4’s training data came from books, Wikipedia, Reddit, Twitter and other public places online. This is the core of the problem; let me explain.
Firstly, let’s cover the elephant in the room. OpenAI is worth around $29 billion thanks to ChatGPT4, yet it would be worth zero without its vast training data. Rightfully so, many Reddit users and other contributors whose data was most likely used by OpenAI are angered, as they feel this is potentially a violation of their copyright over their own creations. Likewise, many artists and photographers whose images were used to create visual AIs like Midjourney are also up in arms about this. But, it goes past just copyright issues. The EU sees this as a data protection breach, which they take very seriously, which is why many AIs are restricted in the EU.
But the issue we are talking about today is filtering this dataset to be good enough for the AI. Take ChatGPT4; if you feed it misspelt, racist, sexist, homophobic, inaccurate or politically swayed content, then it will just replicate this. As you can imagine, there is sadly an awful lot of this type of content online, so this internet scrapping training technique can be highly risky. This is demonstrated by Microsoft’s Tay Twitter chatbot, who was trained on unfiltered tweets, leading to some less than appropriate tweets from Tay, causing the project to be rapidly cancelled.
As such, OpenAI and other AI companies use humans to filter through data and convert it into a format that can be used for training. But, as you can imagine, creating a filtered and refined dataset of 300 billion words is quite the task. So, to avoid overspending, these companies employ foreign workers for as little as $2 per hour to perform these tasks. Which, morally speaking, is highly questionable, but this point deserves its own article.
As these workers are being paid so little, they are looking to employ tools to speed up their work, enabling them to take on more and earn more. A recent experiment by researchers at the École Polytechnique fédérale de Lausanne in Switzerland found that these workers were using AI, like CHatGPT4, to do this.
The researchers hired the same foreign workers and asked them to perform the same dataset filtering and converting process as ChatGPT4 required on 16 medical research papers. This involves taking text, ensuring the text is of a good enough standard, and writing a summary of the text. This summary is used to help the AI understand what the text is about and, in turn, enables it to understand users’ prompts or replies to a user. As such, this summary is vital for AIs like ChatGPT4.
Using their own custom-made AI text detector (which we will discuss later on), the researchers estimated that 33% to 46% of the summaries submitted by these workers were generated using AI, not a human. This makes sense, as it is effortless to make language model AIs like ChatGPT4 to summarise a body text; just copy and paste it into the system and ask it to summarise it. As such, the workers who are using AI in this way are saving themselves buckets of time, enabling them to take on more work and earn more.
This means that it is likely that most generative AIs (even image AIs, as they too need summary texts to work) are currently being trained on their own outputs! This is extremely detrimental, and many have dubbed it as an AI echo chamber, or AI eating itself alive.
But why is it so bad for the AIs? Well, it means that they will start recognising patterns of AI generative content, not human-made content. This can lead to a rabbit hole of development, in which the AI is optimising itself in a counterproductive way. The patterns it sees within the AI content might also go directly against those it sees in human content, leading to incredibly erratic and unstable outputs, which could render the AI useless!
Now, you might think that OpenAI and others can use AI generator detectors to filter out this tainted data and ensure their AI stays squeaky clean. After all, these researchers did the same to identify which foreign works might have used AI. But, as the researchers had a specific request (i.e. summarising medical papers), it was easy to write a program that could detect such AI-generated content from up-to-date language tools such as ChatGPT4. If you were to broaden the requirements, it would become considerably harder to get accurate detection. This is why many AI detection software have huge issues with false positives, which are already landing students in hot water for no reason.
So, even if OpenAI used AI detection software to filter out potentially AI-generated data points, it would still severely affect the neural network. This is because the AI detector’s false positives will skew the dataset, hiding some patterns which the detector thinks are patterns made by an AI that is actually from a human, from the neutral network. This, in turn, will damage the effectiveness of the AI.
So, as it stands, the AI boom might be approaching a flashpoint where these models can’t avoid consuming their own output, leading to a gradual decline in their effectiveness. This will only be accelerated as AI-generated content perfuses the internet over the coming years, making it harder and harder to source genuine human-made content.
There is a way out of this, though. But AI companies won’t like it. Rather than short-changing those providing training data, which is the root cause of this mess, pay them correctly. If an AI company paid me to write and summarise my articles so that they could be submitted into their training database while being monitored to ensure all of it was genuine human content, I would definitely consider it. Particularly if the pay was adequate. But, I doubt OpenAI or any other AI company is willing to do this, as it will rob their profit margins. Instead, they will carry on doing this insane shortcut, which could render their very product useless.
Thanks for reading! Content like this doesn’t happen without your support. So, if you want to see more like this, don’t forget to Subscribe and follow us on Google News, Flipboard, Facebook, Instagram, LinkedIn, and Twitter, or hit the share button below.
Sources: Decrypt, Science Focus, The Register, SAS.com, New Scientist, Time.com, TechCrunch, GPTblogs, The National News, The Guardian, ARXIV