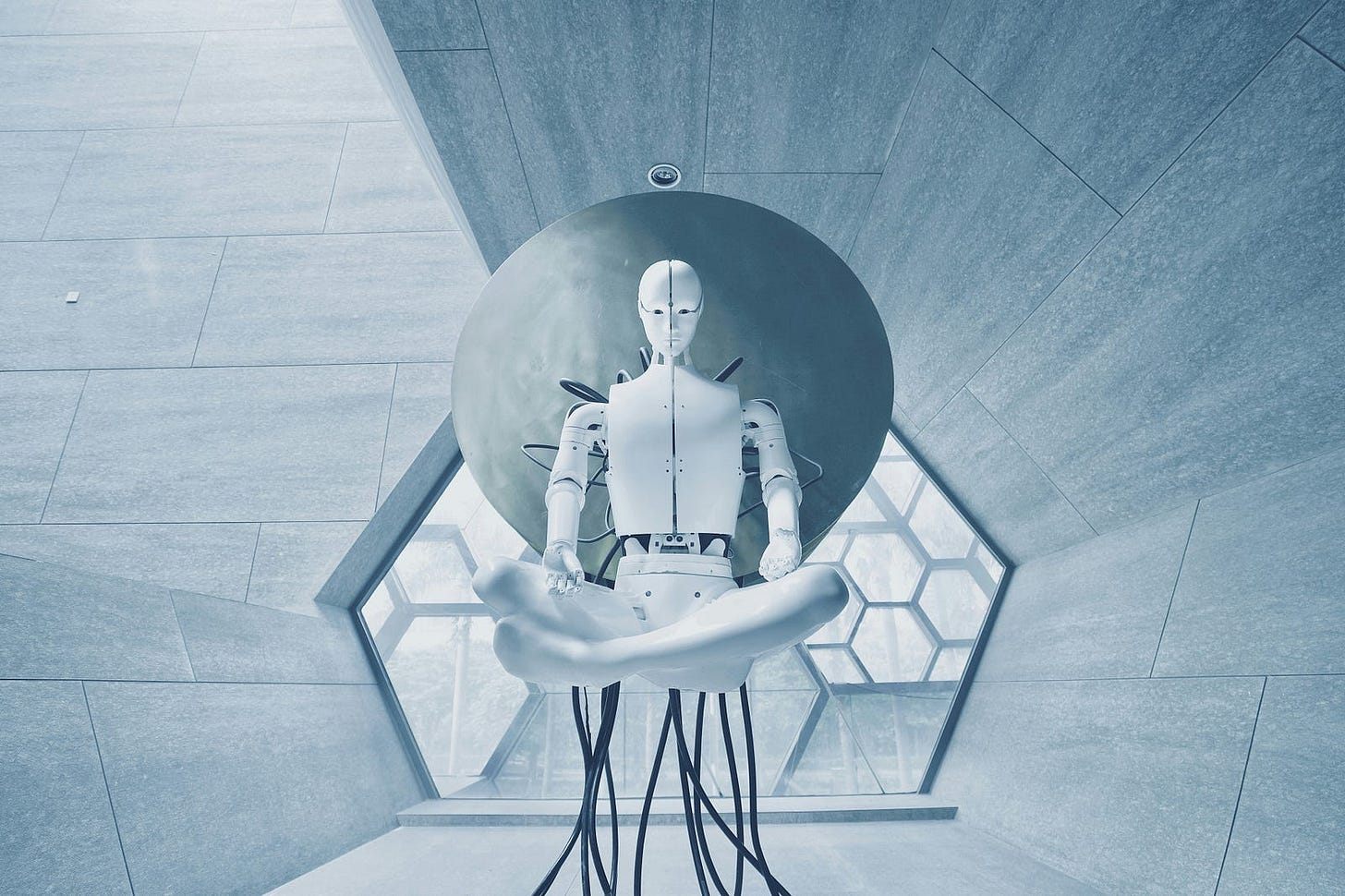
About a month ago, Musk said on an X livestream, “My guess is that we’ll have AI that is smarter than any one human probably around the end of next year”, and this irked me. Ever since, I’ve been mulling over how I can respond to such a deceitful claim. I’m not going to call Musk a con artist or a pathological liar, but I’m going to get damn close. Every aspect of that statement is hollow, misleading and just straight-up bullshit. It fundamentally misrepresents what AI actually is whilst being so ambiguous you could drive a rusty run-away Cybertruck through it. What’s worse, other AI leaders are following in Musk’s footsteps with similar outlandish false predictions and claims. So, here is why you should disregard anyone who claims AI will have superhuman intelligence.
Let’s start with one of the core issues of measuring intelligence. It’s a purely objective measure, making Musk’s statement completely ambiguous without defining parameters. We can’t directly quantitatively measure intelligence (in us or AI), so instead, we measure capabilities. That is why IQ tests measure language, mathematics, reasoning, and spatial comprehension; the test is trying to gauge your abilities in these different areas. But this, in turn, means what capabilities you measure and how you measure them can have a massive impact on the results.
For example, take the classic trope of which is more intelligent, a monkey or a fish. The monkey is obviously the most intelligent if we measure their intelligence based on their ability to climb a tree. But if we measure it based on their ability to navigate 3D space whilst swimming in mirky water, it would be the fish.
Now, some AIs can perform highly constrained tasks better than we humans. There have been multiple AIs that can detect breast cancer from breast screens at a higher rate than doctors. If this was the only capability you measured, you could argue that this makes this AI superhumanly intelligent. Now, this AI obviously doesn’t have higher intelligence than humans. So, because we can’t directly measure intelligence and instead measure capability, you have to be incredibly specific about what you mean by superhuman intelligence; otherwise, such claims are utterly meaningless.
But even if Musk clarified exactly what capabilities he wanted to measure, this statement is still misleading. You see, Artificial Intelligence (AI) is not intelligent in any way, shape, or form.
AI doesn’t actually understand things. It doesn’t build up insights and frameworks to get a deeper understanding of “why”, which is a core part of intelligence and cognition. Instead, AI is purely a mathematical statistical model. That breast cancer AI doesn’t actually understand what cancer is, why it starts, or how it spreads; it has just been fed a tonne of previous screening data and been told which have cancer and which don’t. It finds trends in this data and then compares it to new screens it’s given, and delivers a positive or negative result. ChatGPT works in the same way. It doesn’t actually understand how language works or the meaning of what it is saying; instead, it figures out what statistically should be the next word based on past data it has processed. ChatGPT is basically a super advanced version of predictive text, not a form of intelligence.
This is why AI can work really well in confined and constrained applications, where no novel issues or new variables are introduced, as these can completely derail these statistical models, leading to undesirable or false outputs. For example, breast screening AI can’t work on other similar forms of cancer, even if they start, function and grow the same.
But, thanks to our intelligence, we can cope in unconstrained situations with plenty of novel issues and new variables. You see, we build up understanding and frameworks of why and how things work. This allows us to successfully apply it to entirely new scenarios rather than just applying unrelated and useless statistics. Not only that, but this can also help us recognise when we have got things wrong, as we can compare the outcome of our actions to this deeper level of understanding, and if it doesn’t match up, attempt to resolve these issues. AI can’t do this, as its statistical model can’t recognise mistakes, as it has no understanding of what the “correct” outcome should be, and its actions will always be statistically the right move, no matter the outcome. All of this together also allows us intelligent beings to rapidly learn new tasks, concepts, and skills with very little information.
This is why humans can drive relatively safely after a few hours of practice. In contrast, a self-driving AI trained on millions of hours of driving data and with far more environmental sensors than a human can barely navigate our streets effectively or safely. We have developed an understanding of why we drive like this and built up a framework of the rules of the road. This allows us to drive on roads we have never been to before and react to difficult novel traffic or environmental challenges (mostly) safely, all while following these rules of the road. Meanwhile, self-driving AI, which has no such deep understanding, simply can’t cope with these variables and fails, causing it to suddenly drive into bridges for no reason, drive at full speed through intersections or manoeuvre to run over cyclists (all genuine examples of what self-driving cars have done in the past 3 years).
You see, AI is a misnomer. It simply isn’t intelligent. No matter how advanced you make it, how much data you train it on, or how accurate you can make it, it simply won’t achieve this ability to cognitively understand.
But even if it was intelligent, it’s hitting a brick wall of diminishing returns.
I covered this a few days ago, but here is a quick summary. Making AIs more accurate and capable requires training them on ever large datasets. However, we are getting to a point of diminishing returns. Ten years ago, doubling the dataset size would make a top-of-the-line AI around 5–10% more accurate. However, increasing the performance of today’s cutting-edge AI by the same amount requires a dataset that is hundreds or thousands of times larger. However, the computational power required to train these AIs on this data increases exponentially with the dataset size. We are now rapidly approaching the point where if AI is going to get even marginally better, it will require such a vast amount of energy and computational power that it will be physically impossible. Even OpenAI’s Sam Altman has admitted this is a huge problem and that they need an energy breakthrough like fusion to enable the next generation of AI.
So even if Musk’s statement was actually a poetic way of saying AI will take huge leaps forward over the next year, it is still demonstrably false!
You see, AI isn’t intelligent, and for us to create computer programs that have actual intelligence and develop understanding, frameworks and the associated cognition that goes with this requires an entirely different approach. In fact, this approach has to be so starkly different that it can’t be called AI, as it likely will use different statistical models and program architecture. Sadly, we do not have the first clue how to create a program capable of this, and likely won’t for decades to come, as we have yet to even find the fundamental foundations of such a program. So, anyone claiming AI will be superhuman is selling you snake oil. It’s smoke and mirrors intended purely to line their pockets with your money. Musk owns billions of dollars of shares in Tesla that are artificially inflated thanks to their perceived down-the-line potential of AI self-driving cars. Statements like this pay him millions, despite his self-driving AI being demonstrably dangerous and less capable than humans.
Basically, please don’t fall for this glass bovine excrement. I.e. transparent bullshit.
Thanks for reading! Content like this doesn’t happen without your support. So, if you want to see more like this, don’t forget to Subscribe and follow me on BlueSky or X and help get the word out by hitting the share button below.
Sources: The Guardian, Planet Earth & Beyond, Adcock, Yardeni, How To Learn Machine Learning, The Guardian, Pub Med, HBR, BBC