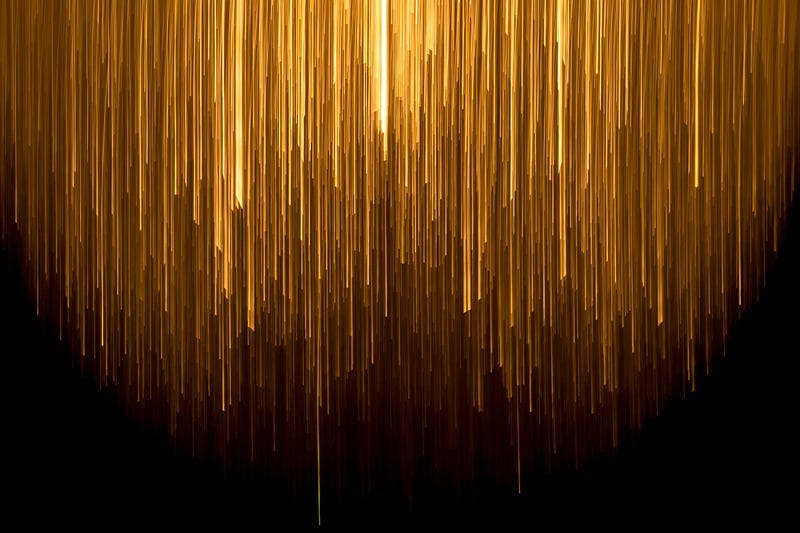
The AI bubble is built entirely on the belief that AI will get substantially better over time. That’s why investors like Microsoft and JP Morgan are pouring tens of billions of dollars into AI companies. Not because their current AIs are especially good but because their future models are meant to be so capable that they could replace vast swathes of workers and make a tonne of money in the process, and they want a slice of that future profit and market control. However, this potentially economy-wrecking gamble is based on shaky ground. You see, it is far from certain that these AIs will get better. In fact, not only is there evidence they can’t get much better than they already are, but a new study shows that AI is actually getting worse!
To understand this study, we first need to get our heads around the two ways you can make AI better: scaling up and shaping up.
In recent years, scaling up has been the method most discussed and the one most heavily used. This involves gathering more training data and using more computational power to “train” the AI (in other words, letting the AI process the data and learn its trends). The other method is shaping up. This involves fine-tuning the AI on the data it already has against human feedback on its performance, effectively making it more efficient with what it already has.
As I have covered before, the AI industry is currently butting up against the limits of the scaling-up method. In short, for AI to keep improving at the same rate, the data, infrastructure, and power usage have to increase exponentially. Because AI companies are already miles away from being profitable, this is simply not a feasible option moving forward (to read more on this, click here).
As such, many are turning to the shaping-up method. This is best exemplified by OpenAI’s o1, or “Strawberry,” model, which doesn’t use more training data than its predecessor (ChatGPT-4o), but instead has an optimised interface that automates a successful prompting technique called chain-of-thought (to read more about o1, and how it achieves this, click here). This enables them to produce “better” AIs without having to splash out an extraordinary amount of cash.
But it seems both methods are reaching their limits and are failing to produce AIs that are functionally better.
This insight came from José Hernández-Orallo, Polytechnic University of Valencia, Spain. He and his colleagues examined the performance of these LLM (Large Language Model) AIs as they improved through scaling up and shaping up. They did this by comparing the latest models from OpenAI, Meta, and BLOOM with their predecessors by giving them tasks ranging from arithmetic problems, solving anagrams, geographical questions, scientific challenges, and pulling out information from disorganised lists. The results are fascinating.
They found that scaling up and shaping up can make these AIs better at answering tricky questions, such as rearranging anagrams. But these AIs actually got worse at solving basic problems. For example, they failed to answer simple arithmetic like addition.
And it isn’t just Hernández-Orallo who has noticed this. A study from the University of California, Berkeley found something similar with GPT-4 and its predecessor GPT-3.5. What’s more, if you Google “AI got worse,” you will find hundreds, possibly even thousands, of articles and posts decrying how new models are far worse at even simple tasks than the previous generation.
So, what’s happening here? Well, both scaling up and shaping don’t magically give these AIs the ability to solve these more complex tasks. In other words, they don’t gain new abilities. Instead, they replace older abilities with new ones, such as losing the ability to answer basic questions.
Like with all AI, we don’t really have many viable options to make these chatbots more broadly applicable; instead, no matter how hard we try, we can only really get them better at specific tasks.
Many thought the shaping-up method could solve this and effectively make the AI able to operate in many “gears,” helping it to be good at solving complex tasks and simple ones (or retain other capabilities it would lose by getting better at others). However, as the shaping-up method was used extensively between the generations in Hernández-Orallo’s study, mainly because they can’t afford to develop solely through scaling up, it shows that this simply hasn’t been the case.
Sadly, there is evidence this inability to broaden its own horizons isn’t just for chatbot AIs. For example, as Tesla’s FSD gets more capable, users report it getting worse at basic tasks such as not running over curbs.
Now, there are some possible solutions, such as running multiple AIs in parallel together, with some being better at some tasks and others at others. This way, the model can appear to have a very broad application, as whatever AI is best suited to your task will be used. But AI companies are already struggling to pay for the development of their current systems, so I doubt this is a feasible approach.
What does this mean for AI? Well, Hernández-Orallo summed it up by saying, “We rely on and we trust them more than we should.” I completely agree; after all, simple mistakes are sometimes the hardest to catch, and as such, his study illuminates a vast flaw in the way we currently use LLM AIs. Not only that, but it also heavily suggests that the paths these AI companies were going to take to bring these industry-disrupting advanced AI models to life aren’t viable. Not only will they actually make the AIs worse at many tasks we need them to be good at, but it doesn’t make AI development any easier or cheaper, meaning they still have no route to profitability or make creating next-generation advanced AI feasible. As such, Hernández-Orallo has also helped highlight how insane the AI bubble actually is.
Thanks for reading! Content like this doesn’t happen without your support. So, if you want to see more like this, don’t forget to Subscribe and help get the word out by hitting the share button below.
Sources: New Scientist, Nature, Will Lockett, Will Lockett, Scientific America, Out Of Spec