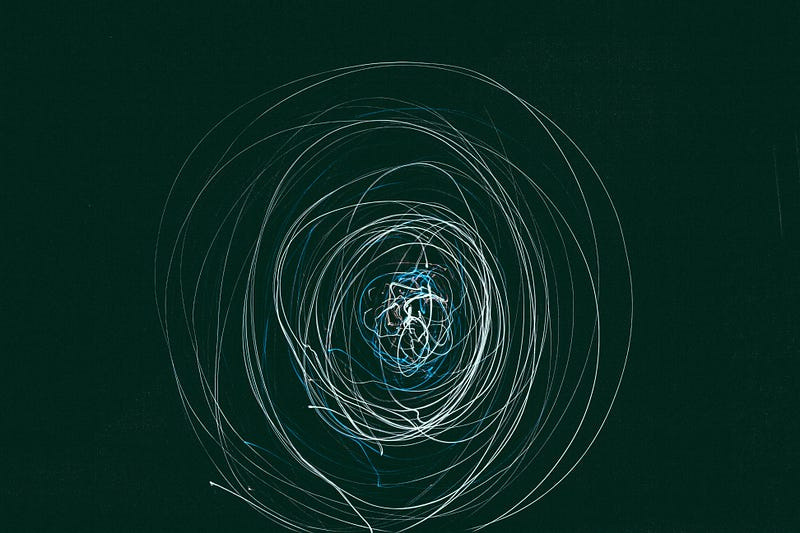
There has been an insane amount of hype surrounding AI over the past few months. Supposedly, Teslas are going to entirely drive themselves in a year or two, AI will be smarter than humans next year, and an army of a billion AI-powered robots will replace human workers by 2040, and that is just the AI promises made by Elon Musk so far this year. The entire AI industry is awash with predictions and promises like this, and it feels like AI development is on an unstoppable exponential trajectory we humans simply can’t stop. However, that is far from the truth. You see, AI is starting to hit a development ceiling of diminishing returns, rendering these extravagant promises utterly hollow. Let me explain.
To understand this problem, we need to understand the basic principles of how AI works. Modern AIs use deep learning algorithms and artificial neural networks to find trends in data. They can then extrapolate from this data or generate new data along the same trend line. This starts by “training” the AI, where a massive amount of data is fed into it for analysis, enabling it to find these trends. After this, the AI can be queried for an output. This basic concept powers computer vision, self-driving cars, chatbots and generative AI. This is a somewhat reductive explanation, but it is all we need to understand for now.
Over the past few years, AIs have become significantly more capable. This has been partly due to better programming and algorithm development. But it is also 90% thanks to the fact that AIs have been trained on significantly larger datasets. This allows them to more accurately understand the trends in the data and, therefore, more accurately generate results. But there is a problem; we are seeing drastically diminishing returns in AI training, both in terms of data and computational power needed.
Let’s start with the data. Let’s say we built a simple computer vision AI designed to recognise dogs and cats, and we trained it using images and videos of 100 dogs and cats, and it can correctly identify them 60% of the time. If we doubled the number of training images and videos to 200, its recognition rate would improve, but only marginally to something like 65%. If we doubled the training images and videos again to 400, its improvement would be even more marginal, to something like 67.5%.
This is partly because when you have a smaller data set, each new training image gives you proportionally more new data to work with than adding a new training image to a larger dataset. However, it is also because AI can quickly make novel connections and trends in a small dataset, as it only has to find a trend that works with a few examples. But as that dataset grows, finding new and novel trends and connections that work for the entire dataset becomes harder and harder. These new trends and connections from larger datasets enable an AI to get better and more capable. As such, we are seeing the amount of training data required to improve an AI by a set amount increase dramatically as we reach a point of diminishing returns with AI training.
But there is another problem. AI training is incredibly computationally hungry. The AI has to compare each individual point of data to every other data point in the set to find these connections and trends. This means that for each bit of data you add to an AI training database, the amount of computational work it takes to train that AI on that database increases exponentially. As such, even if you can acquire the vast amount of data it takes to train these ever-improving AIs, the amount of physical computing power and energy it requires will eventually grow to the point of impossibility.
Sadly, there is evidence that we are at a stage where both the diminishing returns of training dataset growth and the exponential increase in computing power required to use said datasets are enforcing a hard ceiling on AI development.
Take OpenAI’s flagship AI ChatGPT4. Its improvement over ChatGPT3 was smaller than ChatGPT3’s improvement over ChatGPT2, and even though it was more accurate, it still had the same problems of hallucinating facts and lack of understanding as ChatGPT3 did. Now, OpenAI is very tight-lipped on how it develops its AIs, but experts have investigated and found that ChatGPT3 used a training dataset about 78 times larger than ChatGPT2, and ChatGPT4 uses a dataset 571 times larger than ChatGPT3! Yet, despite this considerable up tick in the training dataset size, ChatGPT4 still has significant flaws that significantly limit its use cases. For example, It can’t be trusted to write anything remotely fact-based, as it still makes up facts.
Some estimates put ChatGPT4’s raw training dataset at 45 TB of plaintext. This means that for the next iteration to be as big of an improvement as ChatGPT4 was over ChatGPT3, the training dataset would need to be tens of thousands of TBs. Acquiring and preparing that amount of plaintext data, even with OpenAI’s dubious methods, is simply impractical. However, actually using this dataset to train their AI could use so much energy that the cost renders the AI entirely unviable, even for a non-profit.
That isn’t hyperbole. OpenAI CEO Sam Altman has gone on record saying that an energy breakthrough, like nuclear fusion, is needed to make advanced AI viable. Sadly, even if we do unlock nuclear fusion, it isn’t likely to be cheaper than our current energy this century or possibly even next century. In fact, no form of energy is set to become significantly cheaper than anything we currently have. So, this proposed solution to AI’s energy problem is deeply misleading.
This viewpoint is supported by some very serious studies. One from the University of Massachusetts Amherst looked at the computation and energy costs associated with improving an image recognition AI performance to over 95% accuracy. They found that training such a model would cost $100 billion and produce as much carbon emissions as New York City does in a month. Bearing in mind that this is for an AI that still gets it catastrophically wrong 5% of the time. The study also highlighted that increasing accuracy to 99% would take exponentially more cost and carbon emissions.
This is why Tesla will never develop full self-driving cars with its current approach. Their Autopilot and FSD can only sense the world around them through this type of AI computer vision, and for FSD to become fully self-driving, its image recognition accuracy needs to be approaching 100% accuracy. As this study shows, it could take far more money than even Tesla has to get their AI that good.
In other words, unless the AI industry can find a way to be more efficient with its AI training and its computational load, it won’t be able to break past this limit, and AI development will completely stagnate. Now, possible solutions are on the horizon, such as far more efficient AI hardware incorporating analogue and quantum technologies and new AI architectures that requires significantly smaller training datasets. However, these concepts are still in their infancy and are potentially decades away from being used in the real world.
In short, be prepared for AI to massively fall short of expectations over the next few years.
Thanks for reading! Content like this doesn’t happen without your support. So, if you want to see more like this, don’t forget to Subscribe and follow me on BlueSky or X and help get the word out by hitting the share button below.
Sources: IEEE Spectrum, From The New World, Radio Free Mobile, CDO Trends, Springboard