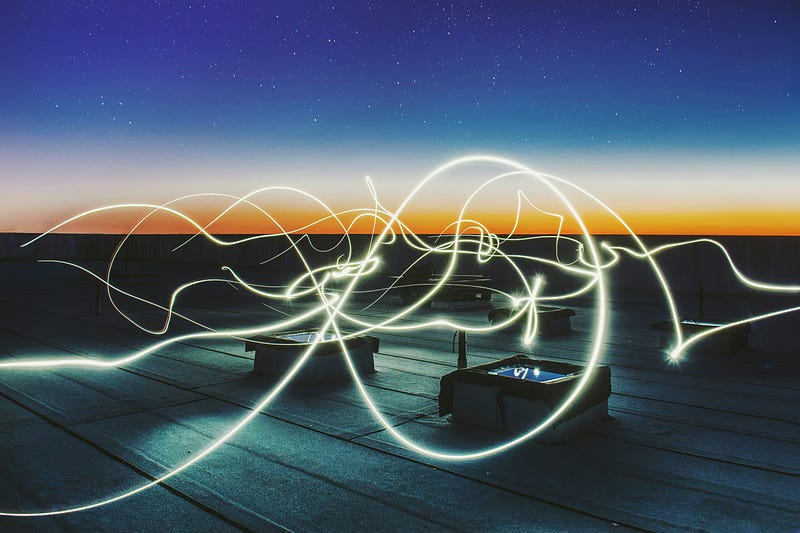
Last month, Open AI CEO Sam Altman attended a World Economic Forum conference. Such an occurrence should be far from noteworthy, except Sam let slip one of AI’s biggest secrets. During one of the many meetings, he warned that the next wave of AI systems will consume vastly more power than expected, and our current energy systems will struggle to cope. He even went as far as to say, “There’s no way to get there [next-gen AI] without a breakthrough.” This marks a profound turning point in the AI hype train, as until now, their energy usage and associated carbon emissions have been the elephant in the room. With this one statement, Altman has painted the elephant pink and got it to do circus tricks for everyone. So, is this energy usage really a problem? What’s its impact? Does Altman have a solution? And can AI be made more energy efficient?
Let’s start with the basics. AI uses energy in two very different ways, training and inference. Training is when you pump a biblical amount of data into an AI and train it on what patterns it should recognise and what it should do with them. Inference is when you take a trained AI and query it for an output.
Of the two, training is by far the most energy-intensive. For example, it has been estimated that Open AI used 1,300 MWh of energy to train its GPT-3 AI, which was a pretty useless text-based generative AI. That is enough energy to power an average US home for 130 years, and US homes are insanely energy inefficient! But the updated and far more capable ChatGPT-4 likely took way more energy to train. GPT-3 only used 17 gigabytes of raw text data to be trained, whereas GPT-4 used 45 gigabytes or 2.6 times more! Therefore, it would have taken way more than 2.6 times more energy to train, as not only does the AI have to shift through 2.6 times more data, but it also has to calculate how all of this extra data relates to each other, find more trends in the data, and compile more complex ‘learnings’. As such, the energy usage of AI training exponentially increases as you increase the volume of data you are training it on.
This is compounded by the fact that we are getting to a point of diminishing returns with AI. Only a few years ago, giving an AI twice the training data would drastically increase its performance once deployed. Now, to get the same increase in performance, you are looking at training the AIs on 10, 100 or 1,000 times more data. This wouldn’t be a problem if our current AIs were good enough, but they aren’t. Generative AI can produce useful work, but is far from reliable or seamless. AI systems are just as woefully unreliable and unpredictable; they can’t even be trusted to drive a car yet. Basically, our AIs need to take massive leaps forward, which means they need a colossal amount of training data and a planet-breaking amount of energy to be trained.
But even after that, AIs are still not energy efficient, as inference also has a considerable energy footprint. It’s been estimated that a search driven by generative AI, a relatively simple task for such an AI, uses four to five times the energy of a conventional web search. Running ChatGPT-4 is also consuming as much energy as 33,000 homes! OpenAI’s GPTs are far from an outlier; every significant AI company, such as Tesla, Facebook, Microsoft, and Google, has the same energy usage issues that are only set to explode over the next few years as the industry heats up.
One researcher has tried to predict this growth and found that by 2027, the global AI industry will consume between 85 and 134 TWhrs yearly. Or, about the same as the Netherlands uses out each year. But remember, it won’t stop there and will only continue to expand exponentially.
This wouldn’t be a problem if our energy was already carbon-neutral. As our energy isn’t, by 2027, the AI sector is set to emit 169.1 million metric tonnes of carbon dioxide each year. It will also push global energy demand (currently 25,000 TWhrs) up by 0.5%, which might not sound significant, but it is! As such, this increase in demand will stifle efforts to phase out carbon-belching energy sources for renewables. Again, remember that this is only for 2027, and this energy demand will skyrocket way past this point.
Sam Altman’s solution? Nuclear fusion! Altman has invested in Helion, which promises to unlock fusion in the very near future. Altman believes this new disruptive source of ultra-clean energy will provide AI with copious amounts of clean energy and power this exaction. However, as any nuclear scientist will tell you, we are still decades away from a nuclear fusion power plant. So Altman’s proposed plan comes across more as PR for his investments…
Some argue that this energy increase is framed wrong, and that AI is actually more energy efficient than letting humans do these tasks. For example, a recent study found that generative AI systems like ChatGPT-4, BLOOM, DALL-E2 and Midjourney produced between 130 and 1500 times less CO2 per page of text generated and between 310 and 2900 times less CO2 per image than their human counterparts. Sadly though, this study didn’t consider social impacts such as professional displacement, legality, or rebound effects for humans, and it weirdly classified the human emissions. So, it isn’t quite comparing apples and apples. It also doesn’t consider that these systems are far from perfect and often need human intervention to check and polish them, or that these models will soon produce far more CO2 as they improve over the coming years.
So, are there any solutions?
Well, yes! These AIs all use a well-established technology known as a neural network. However, some less established but more efficient alternatives, such as spiking neural networks and lifelong learning, don’t require as much training data and train more efficiently. If the industry switched to developing these technologies to be as good as neural networks, it could significantly cut the AI industry’s energy, emissions and even data problems. For example, the AI chatbot BLOOM model 3 uses these techniques and is just as capable as ChatGPT-3 but has a far lower energy demand and carbon footprint.
It’s important to note that these more efficient alternative AI architectures might also be the key to unlocking truly intelligent AI. As I said, our current AI is hitting a diminishing returns issue, so creating intelligent general AI with them might be impossible. On the other hand, these more efficient systems don’t have the same issue and could break past this problem. So, to bring about the AI revolution, not damage the planet, or strain our already stressed energy grids, AI companies must stop developing larger AI models and instead focus on efficiency. At least, that’s a far more fruitful path forward than betting the industry on near-vapourware green energy solutions.
Thanks for reading! Content like this doesn’t happen without your support. So, if you want to see more like this, don’t forget to Subscribe and follow me on BlueSky or X and help get the word out by hitting the share button below.
Sources: Nature, The Conversation, The Verge, Sci Rep, Nexo Code, EPA, Project Pro, IEA, Statista, Statista, Paper Digest